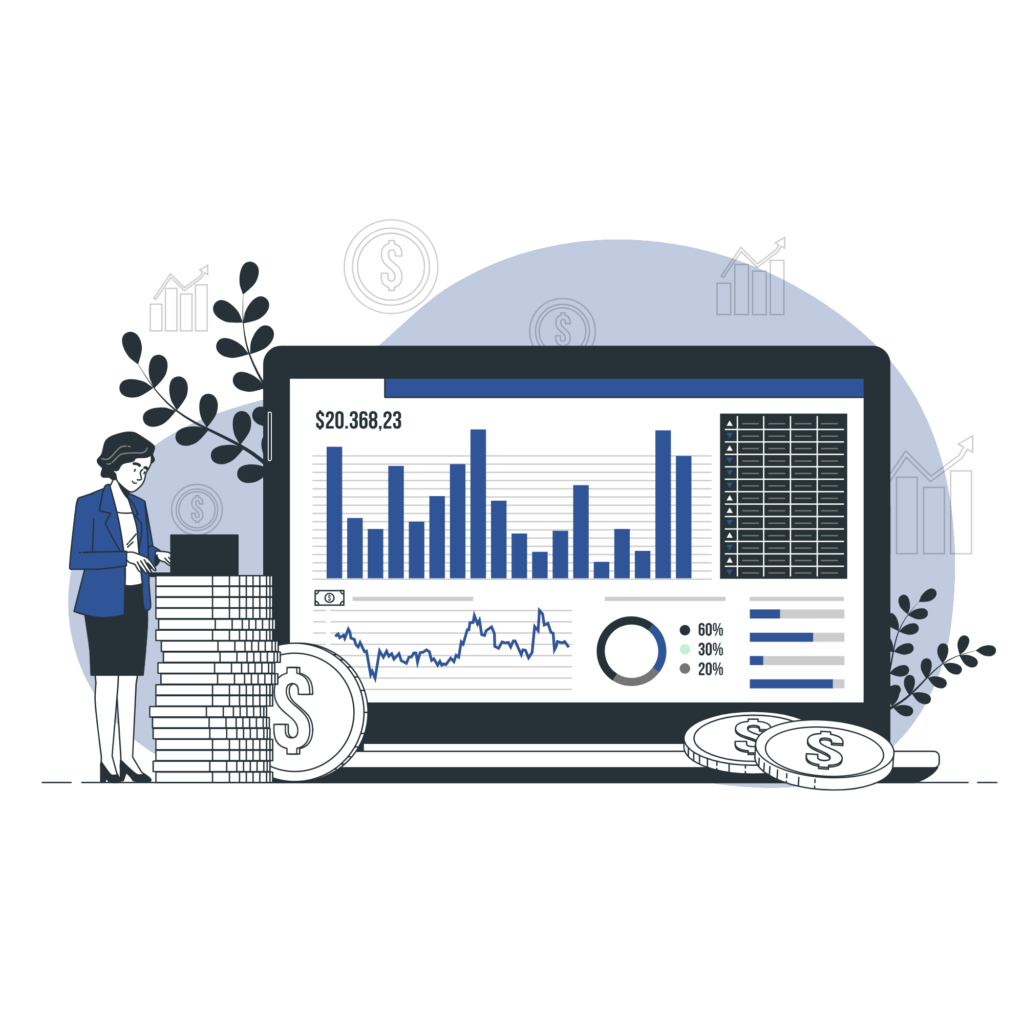
Improvement in the performance of debt collection has become paramount for banks, non-banking financial institutions (NBFCs), and fintech lending entities, reflecting a heightened focus on revenue generation. In the contemporary landscape, which is characterized by its digital nature and emphasis on environmental, social, and governance (ESG) factors, it is imperative to maintain a proactive stance to secure superior recovery rates. An insight suggests that consumer debt has grown to over USD $17.1 trillion in 2023. Reports also predicted a credit card delinquency of over 2.6% in 2023. And the numbers have only been progressing. Debt collection advanced analytics related to debt collection are now regarded as essential by all stakeholders, especially within the realm of lenders. Understanding how lenders behave in a fiscal cycle can help you make decisions faster than your adversaries, facilitating a seamless experience in managing debt collection and recoveries.
This blog explores the intricacies of debt collection, advanced analytics that can revolutionize debt collection practices, offering insights that are beneficial to companies across the spectrum.
The Changing Landscape of Debt Collections
The debt collection landscape has changed significantly in the past decade. Today’s debtors represent a diverse group, with individual financial situations, communication preferences, and habits. In order to improve recovery rates, businesses must interact with consumers in a way that demonstrates greater empathy and respect in light of rising consumer expectations and a global emphasis on the customer experience.
In addition, it is imperative for companies to maintain compliance when they chase outstanding accounts due to the stringency of regulations around debt collection techniques. This shifting terrain implies that a one-size-fits-all approach is no longer practical. Businesses need to adopt a more sophisticated, data-driven technique that uses sophisticated analytics to optimize their debt recovery procedures in order to effectively handle these difficulties.
Credit Ghar has advanced analytical tools based on AI and ML which aids in transforming the journey of debt collection. The key benefits of analytical tools are described in detail along with practical examples showcasing how the analytics can help in debt collection.
1. Personalized Collection Strategies
Incorporating analytics into debt collection involves leveraging customer data to create more effective, customer-centric approaches. This can help debt collectors improve recovery rates, reduce customer churn, and maintain positive relationships.Grouping debtors according to certain attributes, such as payment patterns, credit histories, demographics, or financial well-being, is known as customer segmentation. This enables debt collectors to implement more targeted strategies for every category, guaranteeing that each debtor gets the appropriate information at the appropriate time.This method enables collection agencies to devise tailored and strategic collection for each category.
Personalized outreach or flexible payment arrangements are examples of more proactive actions that may be necessary for high-risk consumers exhibiting indicators of financial distress. However, low-risk clients who have a track record of on-time payments might just need mild reminders or automated follow-ups to pay off their obligation.
Example: Consider a scenario where a company employs analytics to identify that younger customers are more likely to engage with digital platforms, like SMS or email, for reminders, unlike older customers who are more responsive to phone calls. This knowledge empowers the collection team to adjust their approaches, resulting in higher contact rates and overall performance.
2. Predictive Analytics to Identify At-Risk Accounts
Predictive models use historical data and machine learning algorithms to analyze various data points, including payment history, credit scores, employment status, and macroeconomic conditions,Traditional one-size-fits-all approaches are often ineffective in engaging with diverse debtor profiles. NLP can predict the optimal time, communication channel (SMS, email, calls), and the appropriate tone of messaging to enhance engagement with debtors.
By harnessing advanced analytics, business can segment their customer base, understanding unique behaviors, financial situations, and repayment tendencies.Organizations can leverage predictive analytics as an effective mechanism to forecast which accounts are prone to delinquency and those which have a higher likelihood of recovery.This allows collection teams to focus resources on high-risk accounts, offering restructuring options or payment reminders before delinquency occurs.
Example :Imagine a business using predictive analytics to flag accounts that have a high probability of default due to changing economic conditions, such as rising unemployment rates in a debtor’s region. By identifying these at-risk accounts early, the company can offer flexible payment plans or temporary deferrals to help customers stay on track.
3. Enhancing Customer Experience
Traditionally, debt collection was seen as a confrontational and aggressive endeavor, which affected weakend ties between businesses and their customers. However, by utilizing analytics in the collection process, organizations can foster a more empathetic approach—one that not only improves the likelihood of repayment but also protects the customer relationship.
Data analytics enables organizations to gain a deeper insight into a debtor’s financial circumstances, allowing them to customize their communications and proposals accordingly such as a flexible repayment plan,offering such customized solutions can build trust, improve the debtor’s experience, and lead to higher recovery rates.
Example : A customer who has recently faced unemployment may show a greater inclination to interact with a company that provides a grace period or a modified payment plan. By leveraging analytics to recognize this situation, a business can present a suitable offer, thereby facilitating debt recovery while fostering a favorable relationship with the customer.
4.Improving Operational Efficiency
A data-driven strategy is crucial for efficient debt collection, optimizing resource allocation and prioritizing high-potential accounts. This approach optimizes workflows, identifying bottlenecks in the collection process and ensuring agents focus on accounts with high recovery potential, thereby enhancing recovery efficiency
Data analytics significantly improves operational efficiency by automating and optimizing a range of processes. By leveraging data-driven automation in areas such as loan origination, underwriting, and monitoring, the time and effort associated with manual tasks are considerably diminished. This enhancement not only expedites the lending process but also lowers costs, enabling us to provide more competitive loan offerings to our clients.
Example:
As an illustration, a business may use analytics to determine which accounts are likely to repay and give them priority in the collections queue. Because of this, agents are able to work more productively, which lowers operating expenses and increases collection rates.
5. Real-Time Monitoring and Reporting
Real-time reporting and analytics enable collection managers to monitor debt collections strategies in real-time, tracking key performance indicators like recovery rates across different debtor segments, effectiveness of different communication channels like email, sms , phone. And time,how quickly debts are recovered. This allows for agile decision-making and optimizes strategies in the moment, ensuring the recovery process is efficient and effective across different communication channels.
This real-time insight enables businesses to make necessary adjustments and optimize their debt collections strategies.These strategic roadmaps are revolutionizing how organizations manage debt collection.
Example:
A collections team could implement real-time monitoring to assess the response rates across various debtor segments. If they observe that SMS reminders are less effective than email communications, they can swiftly adjust their strategy to focus more on email outreach, thereby enhancing their overall recovery efforts.
6. Compliance and Risk Mitigation
As a debt collection agency, adherence to regulations such as TCPA, FDCPA, Reg-F, GDPR, SARFEASI, and IBC is essential to maintain fair practices in the industry. You are permitted to contact customers within an 11-hour timeframe, specifically from 8 am to 7 pm. Additionally, there are restrictions on the number of calls made to a customer, and it is imperative that the language used remains courteous. Analytics engine guarantees compliance with these regulations and promptly flag any instances of non-compliance issues. Moreover, compliance with these regulatory obligations is automatically guaranteed by the metadata capability of our platform.
Example.
A company might use analytics to monitor compliance with theThe Telephone Collection Practices Act (TCPA), ensuring that all debtor communications are in line with legal requirements. This reduces the risk of fines or lawsuits, protecting the business from costly penalties.
7. Optimizing Third-Party Collection Agency Performance
Many businesses choose to outsource their debt collection efforts to third-party agencies. Even though this is a potentially useful tactic, it’s crucial to make sure these agencies are operating at their best. Analytics can measure variables like recovery rates, time to collect, and cost-effectiveness to provide clear insights into agency performance.
Businesses may make better decisions about which agencies to stick with and which accounts to assign to particular agencies by analyzing these KPIs.
Example:
A business can identify a top-performing agency in a specific geographic region by analyzing data from multiple third-party agencies, enabling the company to allocate more accounts to that agency for maximum recovery.
8. Data-Driven Strategy Adjustments
The debt collection process is dynamic and must change to accommodate shifting consumer preferences, market dynamics, and legislative frameworks. Analytics gives companies the data they need to make quick changes to their collection techniques in real time, allowing them to stay flexible.
Example
Analytics may show that, in a recession or downtime, consumers are more receptive to longer payment terms or smaller payouts; this information enables companies to modify their offers appropriately.
Conclusion
Integrating analytics into debt collection strategies has become a necessity, not a choice, for staying competitive and effective.Banks, NBFCs, and other financial institutions that utilize data-driven insights can optimize their collection processes, improve operational efficiency with compliance and risk mitigation.As companies continue to harness the power of analytics to enhance debt collection strategies, the future of the industry appears more dynamic and optimistic.